導(dǎo)讀
本文整理了pytorch實(shí)現(xiàn)斷電繼續(xù)訓(xùn)練時(shí)需要注意的要點(diǎn),附有代碼詳解。
最近在嘗試用CIFAR10訓(xùn)練分類問題的時(shí)候,由于數(shù)據(jù)集體量比較大,訓(xùn)練的過(guò)程中時(shí)間比較長(zhǎng),有時(shí)候想給停下來(lái),但是停下來(lái)了之后就得重新訓(xùn)練,之前師兄讓我們學(xué)習(xí)斷點(diǎn)繼續(xù)訓(xùn)練及繼續(xù)訓(xùn)練的時(shí)候注意epoch的改變等,今天上午給大致整理了一下,不全面僅供參考
Epoch: 9 | train loss: 0.3517 | test accuracy: 0.7184 | train time: 14215.1018 sEpoch: 9 | train loss: 0.2471 | test accuracy: 0.7252 | train time: 14309.1216 sEpoch: 9 | train loss: 0.4335 | test accuracy: 0.7201 | train time: 14403.2398 sEpoch: 9 | train loss: 0.2186 | test accuracy: 0.7242 | train time: 14497.1921 sEpoch: 9 | train loss: 0.2127 | test accuracy: 0.7196 | train time: 14591.4974 sEpoch: 9 | train loss: 0.1624 | test accuracy: 0.7142 | train time: 14685.7034 sEpoch: 9 | train loss: 0.1795 | test accuracy: 0.7170 | train time: 14780.2831 s絕望!!!!!訓(xùn)練到了一定次數(shù)發(fā)現(xiàn)訓(xùn)練次數(shù)少了,或者中途斷了又得重新開始訓(xùn)練
一、模型的保存與加載
PyTorch中的保存(序列化,從內(nèi)存到硬盤)與反序列化(加載,從硬盤到內(nèi)存)
torch.save主要參數(shù):obj:對(duì)象 、f:輸出路徑
torch.load 主要參數(shù) :f:文件路徑 、map_location:指定存放位置、 cpu or gpu
模型的保存的兩種方法:
1、保存整個(gè)Module
torch.save(net, path)
2、保存模型參數(shù)
state_dict = net.state_dict()torch.save(state_dict , path)
二、模型的訓(xùn)練過(guò)程中保存
checkpoint = { "net": model.state_dict(), 'optimizer':optimizer.state_dict(), "epoch": epoch }
將網(wǎng)絡(luò)訓(xùn)練過(guò)程中的網(wǎng)絡(luò)的權(quán)重,優(yōu)化器的權(quán)重保存,以及epoch 保存,便于繼續(xù)訓(xùn)練恢復(fù)
在訓(xùn)練過(guò)程中,可以根據(jù)自己的需要,每多少代,或者多少epoch保存一次網(wǎng)絡(luò)參數(shù),便于恢復(fù),提高程序的魯棒性。
checkpoint = { "net": model.state_dict(), 'optimizer':optimizer.state_dict(), "epoch": epoch } if not os.path.isdir("./models/checkpoint"): os.mkdir("./models/checkpoint")torch.save(checkpoint,'./models/checkpoint/ckpt_best_%s.pth'%(str(epoch)))
通過(guò)上述的過(guò)程可以在訓(xùn)練過(guò)程自動(dòng)在指定位置創(chuàng)建文件夾,并保存斷點(diǎn)文件
三、模型的斷點(diǎn)繼續(xù)訓(xùn)練
if RESUME: path_checkpoint = "./models/checkpoint/ckpt_best_1.pth" # 斷點(diǎn)路徑 checkpoint = torch.load(path_checkpoint) # 加載斷點(diǎn) model.load_state_dict(checkpoint['net']) # 加載模型可學(xué)習(xí)參數(shù) optimizer.load_state_dict(checkpoint['optimizer']) # 加載優(yōu)化器參數(shù) start_epoch = checkpoint['epoch'] # 設(shè)置開始的epoch
指出這里的是否繼續(xù)訓(xùn)練,及訓(xùn)練的checkpoint的文件位置等可以通過(guò)argparse從命令行直接讀取,也可以通過(guò)log文件直接加載,也可以自己在代碼中進(jìn)行修改。關(guān)于argparse參照我的這一篇文章:
HUST小菜雞:argparse 命令行選項(xiàng)、參數(shù)和子命令解析器
https://zhuanlan.zhihu.com/p/133285373
四、重點(diǎn)在于epoch的恢復(fù)
start_epoch = -1 if RESUME: path_checkpoint = "./models/checkpoint/ckpt_best_1.pth" # 斷點(diǎn)路徑 checkpoint = torch.load(path_checkpoint) # 加載斷點(diǎn) model.load_state_dict(checkpoint['net']) # 加載模型可學(xué)習(xí)參數(shù) optimizer.load_state_dict(checkpoint['optimizer']) # 加載優(yōu)化器參數(shù) start_epoch = checkpoint['epoch'] # 設(shè)置開始的epoch for epoch in range(start_epoch + 1 ,EPOCH): # print('EPOCH:',epoch) for step, (b_img,b_label) in enumerate(train_loader): train_output = model(b_img) loss = loss_func(train_output,b_label) # losses.append(loss) optimizer.zero_grad() loss.backward() optimizer.step()
通過(guò)定義start_epoch變量來(lái)保證繼續(xù)訓(xùn)練的時(shí)候epoch不會(huì)變化
斷點(diǎn)繼續(xù)訓(xùn)練
一、初始化隨機(jī)數(shù)種子
import torchimport randomimport numpy as np def set_random_seed(seed = 10,deterministic=False,benchmark=False): random.seed(seed) np.random(seed) torch.manual_seed(seed) torch.cuda.manual_seed_all(seed) if deterministic: torch.backends.cudnn.deterministic = True if benchmark: torch.backends.cudnn.benchmark = True
關(guān)于torch.backends.cudnn.deterministic和torch.backends.cudnn.benchmark詳見
Pytorch學(xué)習(xí)0.01:cudnn.benchmark= True的設(shè)置
https://www.cnblogs.com/captain-dl/p/11938864.html
pytorch---之cudnn.benchmark和cudnn.deterministic_人工智能_zxyhhjs2017的博客
https://blog.csdn.net/zxyhhjs2017/article/details/91348108
benchmark用在輸入尺寸一致,可以加速訓(xùn)練,deterministic用來(lái)固定內(nèi)部隨機(jī)性
二、多步長(zhǎng)SGD繼續(xù)訓(xùn)練
在簡(jiǎn)單的任務(wù)中,我們使用固定步長(zhǎng)(也就是學(xué)習(xí)率LR)進(jìn)行訓(xùn)練,但是如果學(xué)習(xí)率lr設(shè)置的過(guò)小的話,則會(huì)導(dǎo)致很難收斂,如果學(xué)習(xí)率很大的時(shí)候,就會(huì)導(dǎo)致在最小值附近,總會(huì)錯(cuò)過(guò)最小值,loss產(chǎn)生震蕩,無(wú)法收斂。所以這要求我們要對(duì)于不同的訓(xùn)練階段使用不同的學(xué)習(xí)率,一方面可以加快訓(xùn)練的過(guò)程,另一方面可以加快網(wǎng)絡(luò)收斂。
采用多步長(zhǎng) torch.optim.lr_scheduler的多種步長(zhǎng)設(shè)置方式來(lái)實(shí)現(xiàn)步長(zhǎng)的控制,lr_scheduler的各種使用推薦參考如下教程:
【轉(zhuǎn)載】 Pytorch中的學(xué)習(xí)率調(diào)整lr_scheduler,ReduceLROnPlateau
https://www.cnblogs.com/devilmaycry812839668/p/10630302.html
所以我們?cè)诒4婢W(wǎng)絡(luò)中的訓(xùn)練的參數(shù)的過(guò)程中,還需要保存lr_scheduler的state_dict,然后斷點(diǎn)繼續(xù)訓(xùn)練的時(shí)候恢復(fù)
#這里我設(shè)置了不同的epoch對(duì)應(yīng)不同的學(xué)習(xí)率衰減,在10->20->30,學(xué)習(xí)率依次衰減為原來(lái)的0.1,即一個(gè)數(shù)量級(jí)lr_schedule = torch.optim.lr_scheduler.MultiStepLR(optimizer,milestones=[10,20,30,40,50],gamma=0.1)optimizer = torch.optim.SGD(model.parameters(),lr=0.1) for epoch in range(start_epoch+1,80): optimizer.zero_grad() optimizer.step() lr_schedule.step() if epoch %10 ==0: print('epoch:',epoch)print('learningrate:',optimizer.state_dict()['param_groups'][0]['lr'])
lr的變化過(guò)程如下:
epoch: 10learning rate: 0.1epoch: 20learning rate: 0.010000000000000002epoch: 30learning rate: 0.0010000000000000002epoch: 40learning rate: 0.00010000000000000003epoch: 50learning rate: 1.0000000000000004e-05epoch: 60learning rate: 1.0000000000000004e-06epoch: 70learning rate: 1.0000000000000004e-06
我們?cè)诒4娴臅r(shí)候,也需要對(duì)lr_scheduler的state_dict進(jìn)行保存,斷點(diǎn)繼續(xù)訓(xùn)練的時(shí)候也需要恢復(fù)lr_scheduler
#加載恢復(fù)if RESUME: path_checkpoint = "./model_parameter/test/ckpt_best_50.pth" # 斷點(diǎn)路徑 checkpoint = torch.load(path_checkpoint) # 加載斷點(diǎn) model.load_state_dict(checkpoint['net']) # 加載模型可學(xué)習(xí)參數(shù) optimizer.load_state_dict(checkpoint['optimizer']) # 加載優(yōu)化器參數(shù) start_epoch = checkpoint['epoch'] # 設(shè)置開始的epoch lr_schedule.load_state_dict(checkpoint['lr_schedule'])#加載lr_scheduler #保存for epoch in range(start_epoch+1,80): optimizer.zero_grad() optimizer.step() lr_schedule.step() if epoch %10 ==0: print('epoch:',epoch) print('learning rate:',optimizer.state_dict()['param_groups'][0]['lr']) checkpoint = { "net": model.state_dict(), 'optimizer': optimizer.state_dict(), "epoch": epoch, 'lr_schedule': lr_schedule.state_dict() } if not os.path.isdir("./model_parameter/test"): os.mkdir("./model_parameter/test")torch.save(checkpoint,'./model_parameter/test/ckpt_best_%s.pth'%(str(epoch)))
三、保存最好的結(jié)果
每一個(gè)epoch中的每個(gè)step會(huì)有不同的結(jié)果,可以保存每一代最好的結(jié)果,用于后續(xù)的訓(xùn)練
第一次實(shí)驗(yàn)代碼
RESUME = True EPOCH = 40LR = 0.0005 model = cifar10_cnn.CIFAR10_CNN() print(model)optimizer = torch.optim.Adam(model.parameters(),lr=LR)loss_func = nn.CrossEntropyLoss() start_epoch = -1 if RESUME: path_checkpoint = "./models/checkpoint/ckpt_best_1.pth" # 斷點(diǎn)路徑 checkpoint = torch.load(path_checkpoint) # 加載斷點(diǎn) model.load_state_dict(checkpoint['net']) # 加載模型可學(xué)習(xí)參數(shù) optimizer.load_state_dict(checkpoint['optimizer']) # 加載優(yōu)化器參數(shù) start_epoch = checkpoint['epoch'] # 設(shè)置開始的epoch for epoch in range(start_epoch + 1 ,EPOCH): # print('EPOCH:',epoch) for step, (b_img,b_label) in enumerate(train_loader): train_output = model(b_img) loss = loss_func(train_output,b_label) # losses.append(loss) optimizer.zero_grad() loss.backward() optimizer.step() if step % 100 == 0: now = time.time() print('EPOCH:',epoch,'| step :',step,'| loss :',loss.data.numpy(),'| train time: %.4f'%(now-start_time)) checkpoint = { "net": model.state_dict(), 'optimizer':optimizer.state_dict(), "epoch": epoch } if not os.path.isdir("./models/checkpoint"): os.mkdir("./models/checkpoint")torch.save(checkpoint,'./models/checkpoint/ckpt_best_%s.pth'%(str(epoch)))
更新實(shí)驗(yàn)代碼
optimizer = torch.optim.SGD(model.parameters(),lr=0.1)lr_schedule = torch.optim.lr_scheduler.MultiStepLR(optimizer,milestones=[10,20,30,40,50],gamma=0.1)start_epoch = 9# print(schedule) if RESUME: path_checkpoint = "./model_parameter/test/ckpt_best_50.pth" # 斷點(diǎn)路徑 checkpoint = torch.load(path_checkpoint) # 加載斷點(diǎn) model.load_state_dict(checkpoint['net']) # 加載模型可學(xué)習(xí)參數(shù) optimizer.load_state_dict(checkpoint['optimizer']) # 加載優(yōu)化器參數(shù) start_epoch = checkpoint['epoch'] # 設(shè)置開始的epoch lr_schedule.load_state_dict(checkpoint['lr_schedule']) for epoch in range(start_epoch+1,80): optimizer.zero_grad() optimizer.step() lr_schedule.step() if epoch %10 ==0: print('epoch:',epoch) print('learning rate:',optimizer.state_dict()['param_groups'][0]['lr']) checkpoint = { "net": model.state_dict(), 'optimizer': optimizer.state_dict(), "epoch": epoch, 'lr_schedule': lr_schedule.state_dict() } if not os.path.isdir("./model_parameter/test"): os.mkdir("./model_parameter/test")torch.save(checkpoint,'./model_parameter/test/ckpt_best_%s.pth'%(str(epoch)))
-
數(shù)據(jù)
+關(guān)注
關(guān)注
8文章
7139瀏覽量
89578 -
硬盤
+關(guān)注
關(guān)注
3文章
1317瀏覽量
57492 -
pytorch
+關(guān)注
關(guān)注
2文章
808瀏覽量
13361
原文標(biāo)題:實(shí)操教程|PyTorch實(shí)現(xiàn)斷點(diǎn)繼續(xù)訓(xùn)練
文章出處:【微信號(hào):vision263com,微信公眾號(hào):新機(jī)器視覺】歡迎添加關(guān)注!文章轉(zhuǎn)載請(qǐng)注明出處。
發(fā)布評(píng)論請(qǐng)先 登錄
相關(guān)推薦
Pytorch模型訓(xùn)練實(shí)用PDF教程【中文】
電機(jī)驅(qū)動(dòng)MCU技術(shù)有哪些要點(diǎn)需要注意?
嵌入式系統(tǒng)設(shè)計(jì)時(shí)需要注意的技術(shù)要點(diǎn)和實(shí)現(xiàn)細(xì)節(jié)有哪些?
pytorch模型轉(zhuǎn)換需要注意的事項(xiàng)有哪些?
AVR熔絲位配置的一些要點(diǎn)和需要注意的相關(guān)事項(xiàng)資料下載
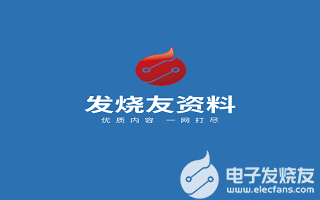
評(píng)論