01
簡(jiǎn)介
《在英特爾開發(fā)者套件上用OpenVINO加速YOLOv8-seg實(shí)例分割模型》介紹了在英特爾者開發(fā)套件上使用 OpenVINO開發(fā)套件部署并測(cè)評(píng) YOLOv8-Seg 的實(shí)例分割模型,本文將介紹在英特爾開發(fā)者套件上使用 OpenVINO2023.0 加速 YOLOv8-Pose 姿態(tài)估計(jì)(Pose Estimation)模型。
請(qǐng)先下載本文的范例代碼倉(cāng),并搭建好 YOLOv8 的OpenVINO 推理程序開發(fā)環(huán)境:
git clone
https://gitee.com/ppovnuc/yolov8_openvino.git
02
導(dǎo)出 YOLOv8-Pose 姿態(tài)估計(jì) OpenVINO IR 模型
YOLOv8-Pose 的姿態(tài)估計(jì)模型有5種,在 COCOKeypoints 數(shù)據(jù)集完成訓(xùn)練,如下表所示。
COCOKeypoints 數(shù)據(jù)集請(qǐng)見:
http://cocodataset.org/
首先使用命令:
yolo export model=yolov8n-pose.pt format=onnx
向右滑動(dòng)查看完整代碼
完成 yolov8n-pose.onnx 模型導(dǎo)出,如下圖所示:
然后使用命令:
mo -m yolov8n-pose.onnx --compress_to_fp16
向右滑動(dòng)查看完整代碼
優(yōu)化并導(dǎo)出 FP16 精度的 OpenVINO IR 格式模型,如下圖所示:
03
用 benchmark_app 測(cè)試 yolov8 姿態(tài)估計(jì)模型的推理計(jì)算性能
benchmark_app 是 OpenVINO工具套件自帶的 AI 模型推理計(jì)算性能測(cè)試工具,可以指定在不同的計(jì)算設(shè)備上,在同步或異步模式下,測(cè)試出不帶前后處理的純 AI 模型推理計(jì)算性能。
使用命令:
benchmark_app -m yolov8n-pose.xml -d GPU
向右滑動(dòng)查看完整代碼
獲得 yolov8n-pose.xml 模型在英特爾開發(fā)者套件的集成顯卡上的異步推理計(jì)算性能,如下圖所示:
04
使用 OpenVINO Python API編寫 YOLOv8-Pose 姿態(tài)估計(jì)模型推理程序
用 Netron 打開 yolov8n-seg.onnx 可以看到模型的輸入和輸出:
輸入節(jié)點(diǎn)名字:“images”;數(shù)據(jù):float32[1,3,640,640]
輸出節(jié)點(diǎn)1的名字:“output0”;數(shù)據(jù):float32 [1,56,8400],其中“8400”是指 YOLOv8 的3個(gè)檢測(cè)頭在 imgsz=640 時(shí),有640/8=80,640/16=40,640/32=20,80x80+40x40+20x20=8400個(gè)輸出單元格;“56”指 “Person” 類的中心坐標(biāo) cx,cy,w,h+“Person” 類的置信分?jǐn)?shù)+“Person” 類的17個(gè)關(guān)鍵點(diǎn)([17,3]) = 56。
基于 OpenVINO Python API 的 YOLOv8 實(shí)例分割模型范例程序 yolov8_pose_ov_sync_infer_demo.py 的核心源代碼,如下所示:
# 實(shí)例化Core對(duì)象 core = Core() # 載入并編譯模型 net = core.compile_model(f'{MODEL_NAME}.xml', device_name="GPU") # 獲得模型輸出節(jié)點(diǎn) output_node = net.outputs[0] ir = net.create_infer_request() cap = cv2.VideoCapture("store-aisle-detection.mp4") while True: start = time.time() ret, frame = cap.read() if not ret: break [height, width, _] = frame.shape length = max((height, width)) image = np.zeros((length, length, 3), np.uint8) image[0:height, 0:width] = frame scale = length / 640 blob = cv2.dnn.blobFromImage(image, scalefactor=1 / 255, size=(640, 640), swapRB=True) # 基于OpenVINO實(shí)現(xiàn)推理計(jì)算 outputs = ir.infer(blob)[output_node] outputs = np.array([cv2.transpose(outputs[0])]) rows = outputs.shape[1] # Postprocess boxes = [] scores = [] preds_kpts = [] for i in range(rows): classes_scores = outputs[0][i][4] key_points = outputs[0][i][5:] if classes_scores >= 0.5: box = [ outputs[0][i][0] - (0.5 * outputs[0][i][2]), outputs[0][i][1] - (0.5 * outputs[0][i][3]), outputs[0][i][2], outputs[0][i][3]] boxes.append(box) scores.append(classes_scores) preds_kpts.append(key_points) result_boxes = cv2.dnn.NMSBoxes(boxes, scores, 0.25, 0.45, 0.5) detections = [] for i in range(len(result_boxes)): index = result_boxes[i] box = boxes[index] pred_kpts = preds_kpts[index] detection = { 'class_id': 0, 'class_name': 'person', 'confidence': scores[index], 'box': box, 'scale': scale} detections.append(detection) print(box[0] * scale, box[1] * scale, scale) draw_bounding_box(frame, 0, scores[index], round(box[0] * scale), round(box[1] * scale), round((box[0] + box[2]) * scale), round((box[1] + box[3]) * scale)) draw_key_points(frame, pred_kpts, 0.2, scale)
向右滑動(dòng)查看完整代碼
運(yùn)行結(jié)果,如下圖所示:
05
結(jié)論
英特爾開發(fā)者套件借助 N5105 處理器的集成顯卡(24個(gè)執(zhí)行單元)和 OpenVINO2023.0 ,可以在 YOLOv8-Pose 的姿態(tài)估計(jì)模型上獲得相當(dāng)不錯(cuò)的性能。通過(guò)異步處理和 AsyncInferQueue ,還能進(jìn)一步提升計(jì)算設(shè)備的利用率,提高 AI 推理程序的吞吐量。
-
英特爾
+關(guān)注
關(guān)注
61文章
10009瀏覽量
172346 -
AI
+關(guān)注
關(guān)注
87文章
31538瀏覽量
270358
原文標(biāo)題:在英特爾開發(fā)者套件上用OpenVINO? 2023.0加速YOLOv8-Pose姿態(tài)估計(jì)模型 | 開發(fā)者實(shí)戰(zhàn)
文章出處:【微信號(hào):英特爾物聯(lián)網(wǎng),微信公眾號(hào):英特爾物聯(lián)網(wǎng)】歡迎添加關(guān)注!文章轉(zhuǎn)載請(qǐng)注明出處。
發(fā)布評(píng)論請(qǐng)先 登錄
相關(guān)推薦
基于C#和OpenVINO?在英特爾獨(dú)立顯卡上部署PP-TinyPose模型
SDK3.0中yolov8-pose用onnx轉(zhuǎn)bmodel自動(dòng)killed如何解決?
英特爾推出了OpenVINO
在AI愛克斯開發(fā)板上用OpenVINO?加速YOLOv8分類模型
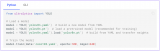
用OpenVINO? C++ API編寫YOLOv8-Seg實(shí)例分割模型推理程序
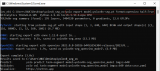
使用英特爾開發(fā)者套件搭建RTMP流媒體服務(wù)器
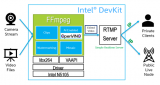
使用OpenVINO優(yōu)化并部署訓(xùn)練好的YOLOv7模型
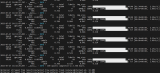
基于OpenVINO在英特爾開發(fā)套件上實(shí)現(xiàn)眼部追蹤
基于英特爾開發(fā)套件的AI字幕生成器設(shè)計(jì)
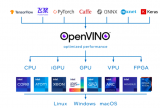
基于英特爾哪吒開發(fā)者套件平臺(tái)來(lái)快速部署OpenVINO Java實(shí)戰(zhàn)
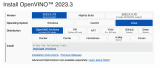
【轉(zhuǎn)載】英特爾開發(fā)套件“哪吒”快速部署YoloV8 on Java | 開發(fā)者實(shí)戰(zhàn)
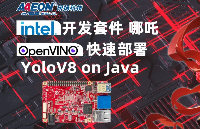
評(píng)論