機器學習的在各領域的廣泛應用也促生其在材料領域的應用。它提供了一種革命性的工具,即能從高維數據中發現數據間的規律。有助于減少計算量從而加速對新材料的探索。對于復雜的數據集,如晶體化合物的數據集,一個至關重要的問題是如何從化學視角為晶體結構構建低維特征。糟糕的特征無法減低數據的復雜性或無法提取晶體的關鍵信息從而導致巨大的預測誤差。為了滿足覆蓋絕大多數晶體結構和組成,特征需要滿足旋轉、平移和尺度不變性。因此完整、精確地數字化描述晶體材料是一項具有挑戰性的任務。
來自北京大學深圳研究生院新材料學院的潘鋒教授和密歇根州立大學數學系的魏國衛教授等提出,原子特殊的持續同調(ASPH)可作為機器學習預測材料形成能的特征,取得了較好的預測效果。不同于傳統拓撲的高級抽象,ASPH能將多尺度幾何信息嵌入拓撲不變量中,能有效地提取幾何空間中的獨立元件、環、空腔等獨特特征。
更具體來說,獨立元件由化學鍵彼此連接,而環和空腔則為多體相互作用。這一發現證明了代數拓撲理論在研究晶體材料中可發揮重要作用。值得注意的是,相比于暨往方法,ASPH對晶體材料形成能的模擬計算有更準確的預測能力,它的適用性可擴展到所有空間群和絕大多數元素。這不僅提供了一種新型的晶體結構描述方法,而且有助于利用計算機輔助設計和新材料發現。
該文近期發表于npj Computational Materials7:28(2021),英文標題與摘要如下,。
Topological representations of crystalline compounds for the machine-learning prediction of materials properties
Yi Jiang, Dong Chen, Xin Chen, Tangyi Li, Guo-Wei Wei & Feng Pan
Accurate theoretical predictions of desired properties of materials play an important role in materials research and development. Machine learning (ML) can accelerate the materials design by building a model from input data. For complex datasets, such as those of crystalline compounds, a vital issue is how to construct low-dimensional representations for input crystal structures with chemical insights.
In this work, we introduce an algebraic topology-based method, called atom-specific persistent homology (ASPH), as a unique representation of crystal structures. The ASPH can capture both pairwise and many-body interactions and reveal the topology-property relationship of a group of atoms at various scales.
Combined with composition-based attributes, ASPH-based ML model provides a highly accurate prediction of the formation energy calculated by density functional theory (DFT). After training with more than 30,000 different structure types and compositions, our model achieves a mean absolute error of 61 meV/atom in cross-validation, which outperforms previous work such as Voronoi tessellations and Coulomb matrix method using the same ML algorithm and datasets.
Our results indicate that the proposed topology-based method provides a powerful computational tool for predicting materials properties compared to previous works.
圖1:以BaTiO3為例,構建晶體拓撲特征的過程
編輯:jq
-
晶體
+關注
關注
2文章
1369瀏覽量
35549 -
拓撲
+關注
關注
4文章
341瀏覽量
29671 -
機器學習
+關注
關注
66文章
8441瀏覽量
133093
原文標題:npj: ML材料性能預測—代數拓撲表達晶體結構
文章出處:【微信號:zhishexueshuquan,微信公眾號:知社學術圈】歡迎添加關注!文章轉載請注明出處。
發布評論請先 登錄
相關推薦
人工智能和機器學習以及Edge AI的概念與應用
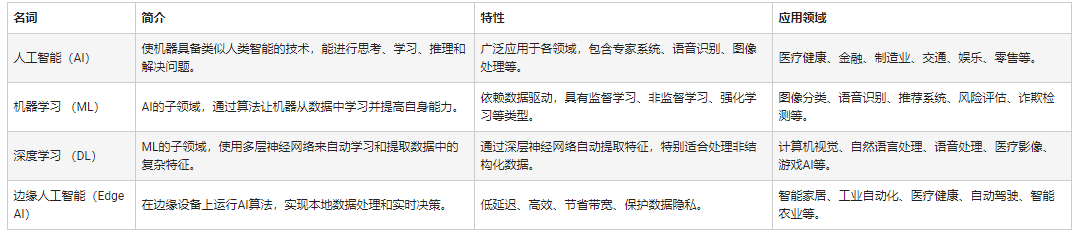
Norflash閃存芯片HT25Q20D廣泛應用在汽車電子領域
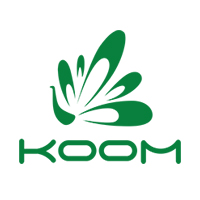
導熱石墨材料在航天電子領域的廣泛應用
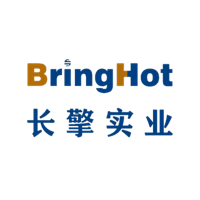
多線示波器的原理和應用領域
可編程交流電子負載各領域的作用
氣體傳感器模塊在應急救援領域的廣泛應用與未來展望
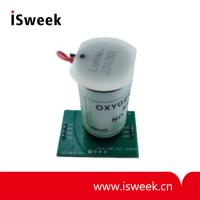
評論