來源:OpenVINO 中文社區
介紹
Retrieval-Augmented Generation (RAG) 系統可以通過從知識庫中過濾關鍵信息來優化 LLM 任務的內存占用及推理性能。歸功于文本解析、索引和檢索等成熟工具的應用,為文本內容構建 RAG 流水線已經相對成熟。然而為視頻內容構建 RAG 流水線則困難得多。由于視頻結合了圖像,音頻和文本元素,因此需要更多和更復雜的數據處理能力。本文將介紹如何利用 OpenVINO 和 LlamaIndex 工具構建應用于視頻理解任務的RAG流水線。
要構建真正的多模態視頻理解RAG,需要處理視頻中不同模態的數據,例如語音內容、視覺內容等。在這個例子中,我們展示了專為視頻分析而設計的多模態 RAG 流水線。它利用 Whisper 模型將視頻中的語音內容轉換為文本內容,利用 CLIP 模型生成多模態嵌入式向量,利用視覺語言模型(VLM)處理檢索到的圖像和文本消息以及用戶請求。下圖詳細說明了該流水線的工作原理。
圖:視頻理解 RAG 工作原理
源碼地址:
https://github.com/openvinotoolkit/openvino_notebooks/tree/latest/notebooks/multimodal-rag
環境準備
該示例基于 Jupyter Notebook 編寫,因此我們需要準備好相對應的 Python 環境。基礎環境可以參考以下鏈接安裝,并根據自己的操作系統進行選擇具體步驟。
https://github.com/openvinotoolkit/openvino_notebooks?tab=readme-ov-file#-getting-started
圖:基礎環境安裝導航頁面
此外本示例將依賴 OpenVINO 和 LlamaIndex 的集成組件,因此我們需要單獨在環境中對他們進行安裝,分別是用于為圖像和文本生成多模態向量的llama-index-embeddings-openvino庫,以及視覺多模態推理llama-index-multi-modal-llms-openvino庫。
模型下載和轉換
完成環境搭建后,我們需要逐一下載流水線中用到的語音識別 ASR 模型,多模型向量化模型 CLIP,以及視覺語言模型模型 VLM。
考慮到精度對模型準確性的影響,在這個示例中我們直接從 OpenVINO HuggingFace 倉庫中,下載轉換以后的 ASR int8 模型。
import huggingface_hub as hf_hub asr_model_id = "OpenVINO/distil-whisper-large-v3-int8-ov" asr_model_path = asr_model_id.split("/")[-1] if not Path(asr_model_path).exists(): hf_hub.snapshot_download(asr_model_id, local_dir=asr_model_path)
而 CLIP 及 VLM 模型則采用 Optimum-intel 的命令行工具,通過下載原始模型對它們進行轉換和量化。
from cmd_helper import optimum_cli clip_model_id = "laion/CLIP-ViT-B-32-laion2B-s34B-b79K" clip_model_path = clip_model_id.split("/")[-1] if not Path(clip_model_path).exists(): optimum_cli(clip_model_id, clip_model_path)
視頻數據提取與處理
接下來我們需要使用第三方工具提取視頻文件中的音頻和圖片,并利用 ASR 模型將音頻轉化為文本,便于后續的向量化操作。在這一步中我們選擇了一個關于高斯分布的科普視頻作為示例(https://www.youtube.com/watch?v=d_qvLDhkg00)。可以參考以下代碼片段,完成對 ASR 模型的初始化以及音頻內容識別。識別結果將被以 .txt 文件格式保存在本地。
from optimum.intel import OVModelForSpeechSeq2Seq from transformers import AutoProcessor, pipeline asr_model = OVModelForSpeechSeq2Seq.from_pretrained(asr_model_path, device=asr_device.value) asr_processor = AutoProcessor.from_pretrained(asr_model_path) pipe = pipeline("automatic-speech-recognition", model=asr_model, tokenizer=asr_processor.tokenizer, feature_extractor=asr_processor.feature_extractor) result = pipe(en_raw_speech, return_timestamps=True)
創建多模態向量索引
這也是整個 RAG 鏈路中最關鍵的一步,將視頻文件中獲取的文本和圖像轉換為向量數據,存入向量數據庫。這些向量數據的質量也直接影響后續檢索任務中的召回準確性。這里我們首先需要對 CLIP 模型進行初始化,利用 OpenVINO 和 LlamaIndex 集成后的庫可以輕松實現這一點。
from llama_index.embeddings.huggingface_openvino import OpenVINOClipEmbedding clip_model = OpenVINOClipEmbedding(model_id_or_path=clip_model_path, device=clip_device.value)
然后可以直接調用 LlamaIndex 提供的向量數據庫組件快速完成建庫過程,并對檢索引擎進行初始化。
from llama_index.core.indices import MultiModalVectorStoreIndex from llama_index.vector_stores.qdrant import QdrantVectorStore from llama_index.core import StorageContext, Settings from llama_index.core.node_parser import SentenceSplitter Settings.embed_model = clip_model index = MultiModalVectorStoreIndex.from_documents( documents, storage_context=storage_context, image_embed_model=Settings.embed_model, transformations=[SentenceSplitter(chunk_size=300, chunk_overlap=30)] ) retriever_engine = index.as_retriever(similarity_top_k=2, image_similarity_top_k=5)
多模態向量檢索
傳統的文本 RAG 通過檢索文本相似度來召喚向量數據庫中關鍵的文本內容,而多模態 RAG 則需要額外對圖片向量進行檢索,用以返回與輸入問題相關性最高的關鍵幀,供 VLM 進一步理解。這里我們會將用戶的提問文本向量化后,通過向量引擎檢索得到與該問題相似度最高的若干個文本片段,以及視頻幀。LlamaIndex 為我們提供了強大的工具組件,通過調用函數的方式可以輕松實現以上步驟。
from llama_index.core import SimpleDirectoryReader query_str = "tell me more about gaussian function" img, txt = retrieve(retriever_engine=retriever_engine, query_str=query_str) image_documents = SimpleDirectoryReader(input_dir=output_folder, input_files=img).load_data()
代碼運行后,我們可以看到檢索得到的文本段和關鍵幀。
圖:檢索返回的關鍵幀和相關文本片段
答案生成
多模態 RAG 流水線的最后一步是要將用戶問題,以及檢索到相關文本及圖像內容送入 VLM 模型進行答案生成。這里我們選擇微軟的 Phi-3.5-vision-instruct 多模態模型,以及 OpenVINO 和 LlamaIndex 集后的多模態模任務組件,完成圖片及文本內容理解。值得注意的是由于檢索返回的關鍵幀往往包含多張圖片,因此這里需要選擇支持多圖輸入的多模態視覺模型。以下代碼為 VLM 模型初始化方法。
from llama_index.multi_modal_llms.openvino import OpenVINOMultiModal vlm = OpenVINOMultiModal( model_id_or_path=vlm_int4_model_path, device=vlm_device.value, messages_to_prompt=messages_to_prompt, trust_remote_code=True, generate_kwargs={"do_sample": False, "eos_token_id": processor.tokenizer.eos_token_id}, )
完成 VLM 模型對象初始化后,我們需要將上下文信息與圖片送入 VLM 模型,生成最終答案。此外在這個示例中還構建了基于 Gradio 的交互式 demo,供大家參考。
response = vlm.stream_complete( prompt=qa_tmpl_str.format(context_str=context_str, query_str=query_str), image_documents=image_documents, ) for r in response: print(r.delta, end="")
運行結果如下:
“A Gaussian function, also known as a normal distribution, is a type of probability distribution that is symmetric and bell-shaped. It is characterized by its mean and standard deviation, which determine the center and spread of the distribution, respectively. The Gaussian function is widely used in statistics and probability theory due to its unique properties and applications in various fields such as physics, engineering, and finance. The function is defined by the equation e to the negative x squared, where x represents the input variable. The graph of a Gaussian function is a smooth curve that approaches the x-axis as it moves away from the center, creating a bell-like shape. The function is also known for its property of being able to describe the distribution of random variables, making it a fundamental concept in probability theory and statistics.”
總結
在視頻內容理解任務中,如果將全部的視頻幀一并送入 VLM 進行理解和識別,會對 VLM 性能和資源占用帶來非常大的挑戰。通過多模態 RAG 技術,我們可以首先對關鍵幀進行檢索,從而壓縮在視頻理解任務中 VLM 的輸入數據量,提高整套系統的識別效率和準確性。而 OpenVINO 與 LlamaIndex 集成后的組件則可以提供完整方案的同時,在本地 PC 端流暢運行流水線中的各個模型。
-
英特爾
+關注
關注
61文章
10043瀏覽量
172580 -
流水線
+關注
關注
0文章
122瀏覽量
26064 -
模型
+關注
關注
1文章
3392瀏覽量
49355 -
OpenVINO
+關注
關注
0文章
104瀏覽量
259
原文標題:開發者實戰|如何利用 OpenVINO? 在本地構建多模態 RAG 應用
文章出處:【微信號:英特爾物聯網,微信公眾號:英特爾物聯網】歡迎添加關注!文章轉載請注明出處。
發布評論請先 登錄
相關推薦
【「基于大模型的RAG應用開發與優化」閱讀體驗】+第一章初體驗
【「基于大模型的RAG應用開發與優化」閱讀體驗】RAG基本概念
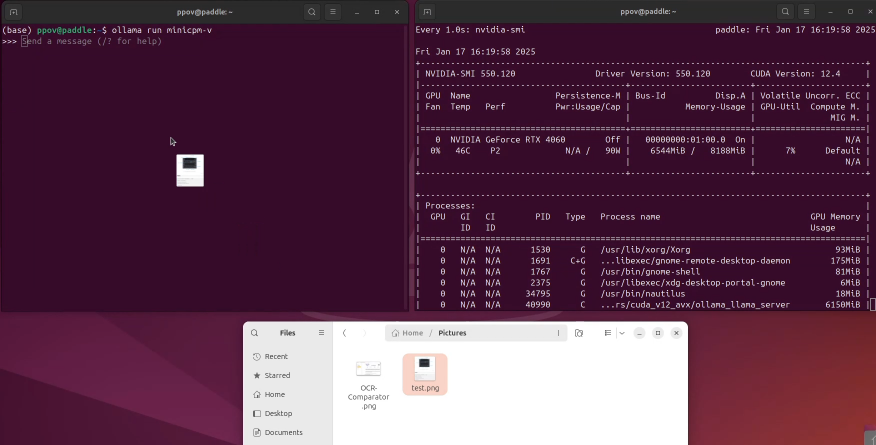
利用OpenVINO工具包檢測汽車品牌
從源代碼構建OpenVINO工具套件時報錯怎么解決?
OpenVINO工具套件是否可以商業化使用?
在Raspberry Pi上從源代碼構建OpenVINO 2021.3收到錯誤怎么解決?
如何使用交叉編譯方法為Raspbian 32位操作系統構建OpenVINO工具套件的開源分發
如何使用Python包裝器正確構建OpenVINO工具套件
永久設置OpenVINO trade Windows reg10的工具套件環境變量
如何利用LLM做多模態任務?
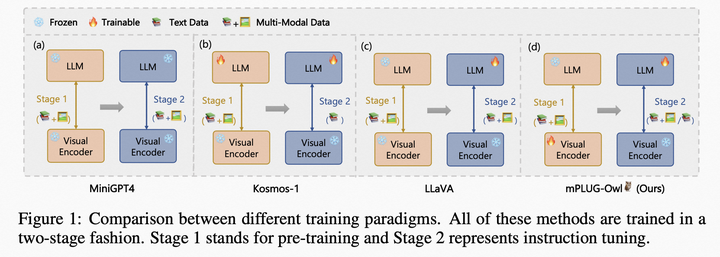
使用OpenVINO和LlamaIndex構建Agentic-RAG系統
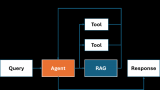
評論