在過去的一年里,人工智能正以越來越快的速度發展,這得益于生成式 AI 模型的引入和從中受益的場景的演變。我們承認這一點,并決定比平時更快地發布新版本的 OpenVINO ,以幫助您獲得新功能!
與之前的版本一樣,在提高性能、增加對新 AI 模型的支持以及構建基礎設施和模型緩存等不同組件方面,我們做了大量工作。對于我們最新的 2023.2 版本,我們做出了一些重大改進,我們將在下面概述。
邊緣文本生成模型的附加性能
在我們的上一個版本 2023.1 中,我們引入了一些更改,以在英特爾 CPU 和 GPU 上運行大語言模型(LLM)。開發者們能夠量化權重為 int8 格式,并將其作為初始步驟在 CPU 上運行。
在 2023.2 版本中,我們進一步優化此工作流程,并引入在 CPU 和集成顯卡上運行權重量化為 int8 和 int4 精度的 LLM 的能力。權重量化直接影響內存帶寬,并幫助模型更快、更高效地執行推理,因為模型消耗的內存更少了,所需的磁盤空間也更少,因此總體上需要的內存帶寬也更少了!
此外,我們的模型轉換和優化工具已經更新,可以幫助您處理模型準備流程。要壓縮模型權重為 int8 和 int4 格式,您可以使用我們的神經網絡壓縮框架(NNCF)工具,該工具適用于 OpenVINO 格式或中間表示(IR)文件。此外,為了獲得具有int4壓縮權重的模型,您可以通過 GPTQ(生成預訓練 transformers 量化)算法來優化轉換模型。實現這一過程的一種方法是通過 Hugging Face AutoGPTQ 實現。
如果你將 Hugging Face 作為模型的來源,你可以使用我們的 optimum-Intel,它集成了 OpenVINO 的優勢。此集成允許您自動壓縮和轉換模型,如我們在下面所示的這樣:
要將模型壓縮到 int8 精度:
#make use of optimum-intel from optimum.intel import OVModelForCausalLM #load pretrained model, convert to OpenVINO representation #and compress weights model = OVModelForCausalLM.from_pretrained("meta-llama/Llama-2-7b", use_cache=True, export=True, load_in_8bit=True) #store OpenVINO IR in a folder model.save_pretrained("./Llama-2-7b")
左滑查看更多
請注意“load_in_8bit”選項,該選項指定應將原始模型壓縮到 int8 精度。對于大于 1B 的模型,默認情況下會啟用此選項。
要將模型壓縮到 int4 精度:
#make use of optimum-intel from optimum.intel import OVModelForCausalLM #explicitly use NNCF for compression from nncf import compress_weights, CompressWeightsMode #load pretrained model, convert to OpenVINO representation model = OVModelForCausalLM.from_pretrained("meta-llama/Llama-2-7b", use_cache=True, export=True, load_in_8bit=False) #perform weights compression using NNCF model.model = compress_weights(model.model, mode=CompressWeightsMode.INT4_SYM, group_size=128, ratio=0.8) #store OpenVINO IR in a folder model.save_pretrained("./Llama-2-7b")
左滑查看更多
請注意,這一次我們沒有使用 HF API 功能,而是直接調用 NNCF 將權重壓縮到 int4。根據模型的不同,您可以更改壓縮參數以獲得更準確的結果。在這種情況下,我們使用對稱量化,組大小為 128 個元素,int4 與 int8 的權重之比為 0.8。您可以查看我們的權重壓縮文檔,以獲得更多詳細信息和壓縮提示。
要轉換使用 AutoGPTQ 優化為 int4 精度的模型,請執行以下操作:
#make use of optimum-intel from optimum.intel import OVModelForCausalLM #load pretrained model, convert to OpenVINO representation #with keeping weights in int4 model = OVModelForCausalLM.from_pretrained("TheBloke/Llama-2-7B-GPTQ", use_cache=True, export=True) #store OpenVINO IR in a folder model.save_pretrained("./Llama-2-7B-GPTQ")
新的生成式 AI
以及更多的 Notebooks 代碼示例!
我們知道,親身體驗最新功能和最先進模型是最好的學習方式。因此,我們的 OpenVINO 團隊非常專注于為 OpenVINO Notebooks 代碼示例帶來新的及備受關注的模型。我們希望展示并鼓勵您立即在您的設備上進行本地實驗,以獲得您所需的性能。以下是我們最近更新或新發布的一些 Notebooks 代碼示例,以幫助您更快地將想法付諸生產。
一些 Jupyter Notebooks 已經更新,以演示 PyTorch 模型在沒有 ONNX 轉換的情況下的轉換和優化,包括以下內容:
PyTorch to OpenVINO
(https://github.com/openvinotoolkit/openvino_notebooks/tree/main/notebooks/102-pytorch-to-openvino )
—— 轉換格式為 torch.nn.Module 以及 torch.jit.ScriptModule 的 PyTorch 模型為 OpenVINO IR 格式
Post-Training Quantization of PyTorch models with NNCF
(https://github.com/openvinotoolkit/openvino_notebooks/blob/main/notebooks/112-pytorch-post-training-quantization-nncf/112-pytorch-post-training-quantization-nncf.ipynb )
—— 將 int8 量化應用于 PyTorch 模型
Quantization of Image Classification Models
(https://github.com/openvinotoolkit/openvino_notebooks/blob/main/notebooks/113-image-classification-quantization/113-image-classification-quantization.ipynb )
—— 將 int8 量化應用于 MobileNet V2 PyTorch 模型
Visual Question Answering and Image Captioning using BLIP and OpenVINO
(https://github.com/openvinotoolkit/openvino_notebooks/tree/main/notebooks/233-blip-visual-language-processing )
—— 優化 BLIP PyTorch 模型
Text-to-Image Generation and Infinite Zoom with Stable Diffusion v2 and OpenVINO
(https://github.com/openvinotoolkit/openvino_notebooks/tree/main/notebooks/236-stable-diffusion-v2 )
—— 在 Stable Diffusion 2.0 流水線中優化模型
Object masks from prompts with SAM and OpenVINO
(https://github.com/openvinotoolkit/openvino_notebooks/tree/main/notebooks/237-segment-anything#object-masks-from-prompts-with-sam--and-openvino )
—— 優化基于 PyTorch 的 Segment Anything Model (SAM) 模型
Optimizing PyTorch models with Neural Network Compression Framework of OpenVINO by 8-bit quantization
(https://github.com/openvinotoolkit/openvino_notebooks/tree/main/notebooks/302-pytorch-quantization-aware-training )
—— PyTorch 模型量化感知訓練(QAT)
我們還加入了一些 notebooks 代碼示例,展示如何轉換和優化模型,包括來自 TensorFlow Hub, TorchVision, and Hugging Face Hub 的模型。
TorchaVision Zoo with OpenVINO
(https://github.com/openvinotoolkit/openvino_notebooks/tree/main/notebooks/125-torchvision-zoo-to-openvino )
—— 下載和直接優化基于 PyTorch 的預訓練模型
Hugging Face Model Hub with OpenVINO
(https://github.com/openvinotoolkit/openvino_notebooks/tree/main/notebooks/124-hugging-face-hub )
—— 學習如何下載和優化 Hugging Face hub 的預訓練模型
TensorFlow Hub models + OpenVINO
(https://github.com/openvinotoolkit/openvino_notebooks/tree/main/notebooks/126-tensorflow-hub )
—— 學習如何下載和優化 TensorFlow Hub 的預訓練模型
Convert Detectron2 Models to OpenVINO
(https://github.com/openvinotoolkit/openvino_notebooks/tree/main/notebooks/123-detectron2-to-openvino )
—— 優化來自 Facebook Research 流行的目標檢測和分割模型
Convert TensorFlow Object Detection and Instance Segmentation Models to OpenVINO
(https://github.com/openvinotoolkit/openvino_notebooks/tree/main/notebooks/120-tensorflow-object-detection-to-openvino)
—— 優化來自于 TensorFlow Hub 的使用 Resnet-50 V1 的 Faster R-CNN
Visual-language assistant with LLaVA and OpenVINO
(https://github.com/openvinotoolkit/openvino_notebooks/tree/main/notebooks/257-llava-multimodal-chatbot )
—— 使用 LLaVA (Large Language and Vision Assistant) 的端到端多模態演示
Subject-driven image generation and editing using BLIP Diffusion and OpenVINO
(https://github.com/openvinotoolkit/openvino_notebooks/tree/main/notebooks/258-blip-diffusion-subject-generation )
—— 優化用于零樣本主題驅動的圖像生成的 BLIP 擴散模型
SoftVC VITS Singing Voice Conversion and OpenVINO
(https://github.com/openvinotoolkit/openvino_notebooks/tree/main/notebooks/262-softvc-voice-conversion#softvc-vits-singing-voice-conversion-and-openvino )
—— 優化以音頻作為輸入的聲音轉換模型 SoftVC 及 VITS
Object segmentations with FastSAM and OpenVINO
(https://github.com/openvinotoolkit/openvino_notebooks/tree/main/notebooks/261-fast-segment-anything )
—— 優化用于目標分割的 Fast Segment Anything Model (FastSAM) 模型
Image Generation with DeciDiffusion
(https://github.com/openvinotoolkit/openvino_notebooks/tree/main/notebooks/259-decidiffusion-image-generation )
—— 優化用于文生圖的 DeciDiffusion 1.0 模型
Document Visual Question Answering Using Pix2Struct and OpenVINO
(https://github.com/openvinotoolkit/openvino_notebooks/tree/main/notebooks/260-pix2struct-docvqa )
—— 利用 OCR 和語言模型進行多模態問答的演示
圖1:文檔視覺問答
最后,我們還提供了幾個具有開箱即用、優化性能的流行的生成式 AI 的 notebooks 代碼示例:
Create an LLM-powered Chatbot using OpenVINO
(https://github.com/openvinotoolkit/openvino_notebooks/tree/main/notebooks/254-llm-chatbot#create-llm-powered-chatbot-using-openvino )
—— 在 CPU 和 GPU 上運行具有 int8 權重壓縮的 Llama2 等聊天機器人,令人印象深刻的是,它將在只有 24GB RAM 的筆記本電腦上運行。
Image generation with Latent Consistency Model and OpenVINO
(https://github.com/openvinotoolkit/openvino_notebooks/tree/main/notebooks/263-latent-consistency-models-image-generation )
—— 用低得多的計算機資源實現卓越的圖像生成
Generate creative QR codes with ControlNet QR Code Monster and OpenVINO
(https://github.com/openvinotoolkit/openvino_notebooks/tree/main/notebooks/264-qrcode-monster )
—— 使用 ControlNet 和 Stable Diffusion 創建您自己的圖形二維碼。
圖2:使用OpenVINO 優化基于大語言模型的聊天機器人
圖3: ControlNet二維碼 Monster 以及 OpenVINO
新的分發渠道
在這個版本中,我們繼續改進您訪問和使用 OpenVINO 進行 AI 應用程序開發的方式,我們已經開發了一個Conan(https://conan.io)軟件包管理器。Conan允許您為大型項目執行包管理,我們很高興看到已經有開發者對此做出的積極回應。
有關如何使用 OpenVINO Conan 軟件包的更多詳細信息,請參閱此處:
https://docs.openvino.ai/2023.1/openvino_docs_install_guides_installing_openvino_conan.html
(復制鏈接到瀏覽器打開)
OpenVINO 是在開源中開發的,一旦在我們的初步測試中驗證了這些功能,我們的最新功能就可以在我們的主分支上獲得。因此,如果您想嘗試新功能,您可以隨時從源代碼構建我們的包。對于 pip 用戶,我們通過引入 openvino-nightly 包來簡化這一點。你可以使用這個每日構建的包來嘗試最新的功能,并在我們的下一個官方版本中預覽一下!
開源貢獻對我們來說很重要!
OpenVINO 已經是一個超過 5 年的開源項目了。最近,我們準備了一系列貢獻任務,通過向 OpenVINO 貢獻,可以更好地幫助社區圍繞人工智能生態系統和開源項目構建知識。這包括支持新的編譯選項和添加更多需要注意的操作等任務。
查看我們在 GitHub 上的鏈接,看看有沒有你感興趣的任務!
如上所述,我們非常感謝迄今為止我們看到的所有被合并進來的開源貢獻。我們還要公開感謝我們最近的一些貢獻者!他們是Siddhant Chauhan、rsa-10、Santhosh Mamidisetti 和 Mahimai Raja J.。由于您的幫助,產品變得更好!
-
人工智能
+關注
關注
1796文章
47683瀏覽量
240313 -
生態系統
+關注
關注
0文章
704瀏覽量
20784 -
開源項目
+關注
關注
0文章
38瀏覽量
7254 -
生成式AI
+關注
關注
0文章
514瀏覽量
548
原文標題:OpenVINO? 2023.2 發布:讓生成式 AI 在實際場景中更易用
文章出處:【微信號:SDNLAB,微信公眾號:SDNLAB】歡迎添加關注!文章轉載請注明出處。
發布評論請先 登錄
相關推薦
生成式AI在制造業的應用現狀和前景展望
使用OpenVINO GenAI API在C++中構建AI應用程序
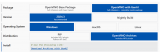
評論