高彈性模量、低重量的SiO2基玻璃的化學設計是一個重要的研究課題。然而,由于彈性模量是原子間鍵和鍵序在不同尺度上的復函數,在合成前很難找到一個根據玻璃成分預測彈性模量的通用表達式。特別是,如何發現新的玻璃成分以獲得高彈性模量和低密度,對當今強化和耐用的SiO2玻璃材料的發展具有重要意義。與晶體材料不同的是,SiO2基玻璃的彈性模量不僅由原子鍵合強度決定,而且還取決于許多其他物理性質在不同尺度的復合函數,如陽離子配位、原子環、鏈、層和多面體原子團簇的形成,甚至是在介觀尺度上的結構組織。此外,氧化物添加劑還引入不同價態的陽離子,不僅改變了陽離子與氧的鍵合強度,而且還改變了網絡聚合的程度。因此,SiO2玻璃的彈性模量是氧化物化學成分的復雜函數,很難開發理論模型來探索多種添加劑氧化物的混合效應,很難直接用于發現新的玻璃成分,很難定量地解釋與玻璃化學有關的優化結果。
來自Michigan大學的Liang Qi (齊亮)教授等,將機器學習(ML)方法與高通量MD模擬相結合,建立了一個定量準確的模型,根據玻璃成分,預測SiO2基玻璃的密度和彈性模量。研究了Li2O、Na2O、K2O、CaO、SrO、Al2O3、Y2O3、La2O3、Ce2O3、Eu2O3、Er2O3、B2O3和ZrO2等13種添加劑的效果。訓練集是使用MD模擬生成的,以均勻采樣組成二元和三元系統的一部分的密度和彈性。從用于MD模擬的力場勢和元素摩爾分數中精心構造了一組描述符,以囊括其物理和成分信息。通過大量的模擬和實驗數據的驗證,模型不僅在訓練集的組成范圍內,而且在訓練集的高維組成空間內,都對SiO2基玻璃的密度和彈性模量具有很好的預測能力。所建立的ML模型可用于快速篩選玻璃組成特性,從而對一般多組分玻璃體系的密度和彈性特性進行有效的預測,特別是未探索的組成區域。
該文近期發表于npj Computational Materials 6: 25 (2020)
Predicting densities and elastic moduli of SiO2-based glasses by machine learning
Yong-Jie Hu, Ge Zhao, Mingfei Zhang, Bin Bin, Tyler Del Rose, Qian Zhao, Qun Zu, Yang Chen, Xuekun Sun, Maarten de Jong and Liang Qi
Chemical design of SiO2-based glasses with high elastic moduli and low weight is of great interest. However, it is difficult to find a universal expression to predict the elastic moduli according to the glass composition before synthesis since the elastic moduli are a complex function of interatomic bonds and their ordering at different length scales. Here we show that the densities and elastic moduli of SiO2-based glasses can be efficiently predicted by machine learning (ML) techniques across a complex compositional space with multiple (》10) types of additive oxides besides SiO2. Our machine learning approach relies on a training set generated by high-throughput molecular dynamic (MD) simulations, a set of elaborately constructed descriptors that bridges the empirical statistical modeling with the fundamental physics of interatomic bonding, and a statistical learning/predicting model developed by implementing least absolute shrinkage and selection operator with a gradient boost machine (GBM-LASSO)。 The predictions of the ML model are comprehensively compared and validated with a large amount of both simulation and experimental data. By just training with a data set only composed of binary and ternary glass samples, our model shows very promising capabilities to predict the density and elastic moduli for k-nary SiO2-based glasses beyond the training set. As an example of its potential applications, our GBM-LASSO model was used to perform a rapid and low-cost screening of many (~105) compositions of a multicomponent glass system to construct a compositional-property database that allows for a fruitful overview on the glass density and elastic properties.
責任編輯:pj
-
機器學習
+關注
關注
66文章
8438瀏覽量
132954 -
玻璃材料
+關注
關注
0文章
2瀏覽量
5783 -
SiO2
+關注
關注
0文章
22瀏覽量
8541
發布評論請先 登錄
相關推薦
利用Phase Lab鎳基摩爾體積數據庫實現材料物性參數的精準預測
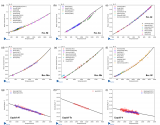
玻璃基芯片先進封裝技術會替代Wafer先進封裝技術嗎
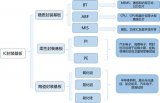
優化銅互連結構的熱應力分析與介電材料選擇
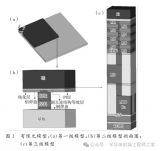
SiO2薄膜的刻蝕機理
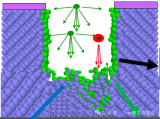
影響應變計靈敏系數的因素有哪些?
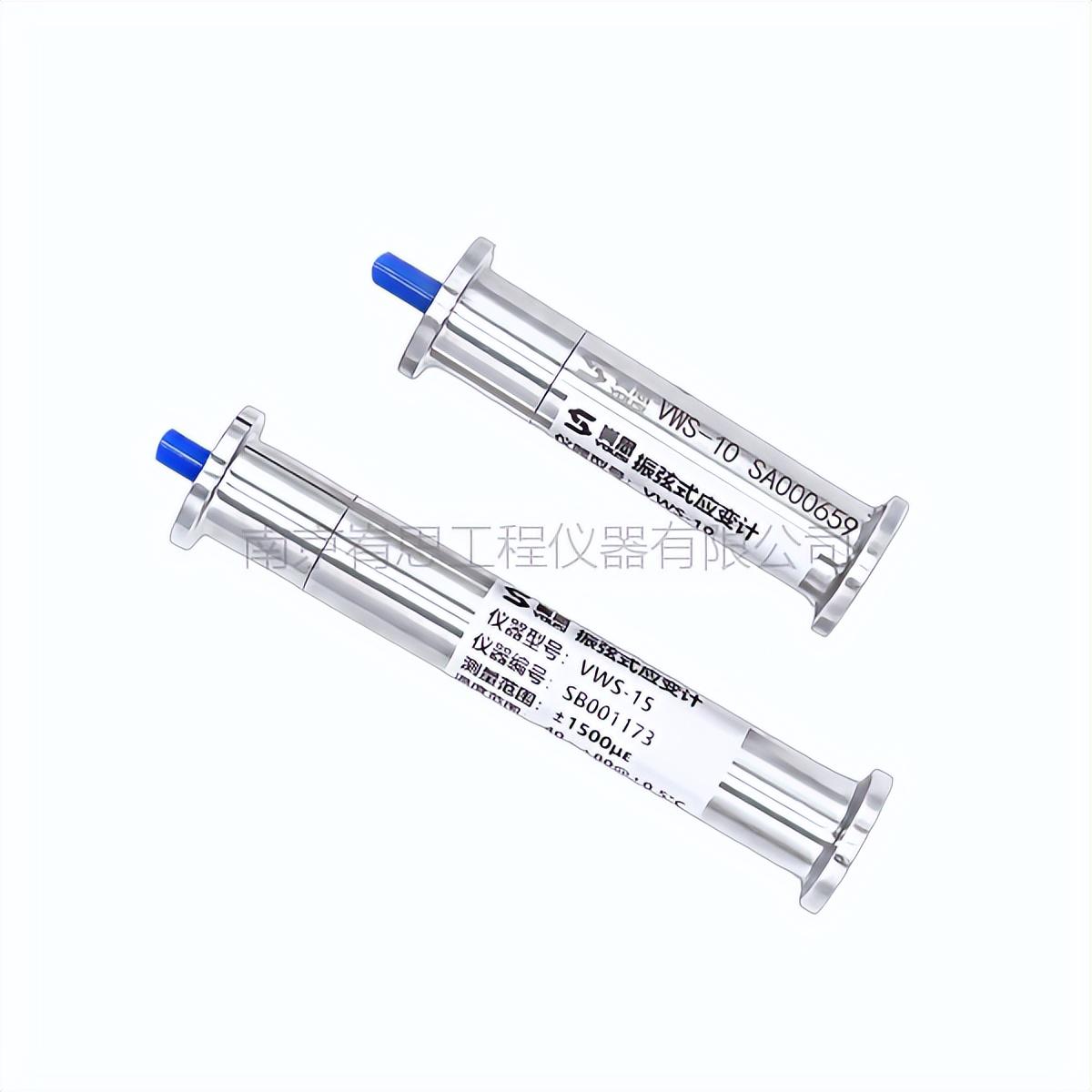
評論